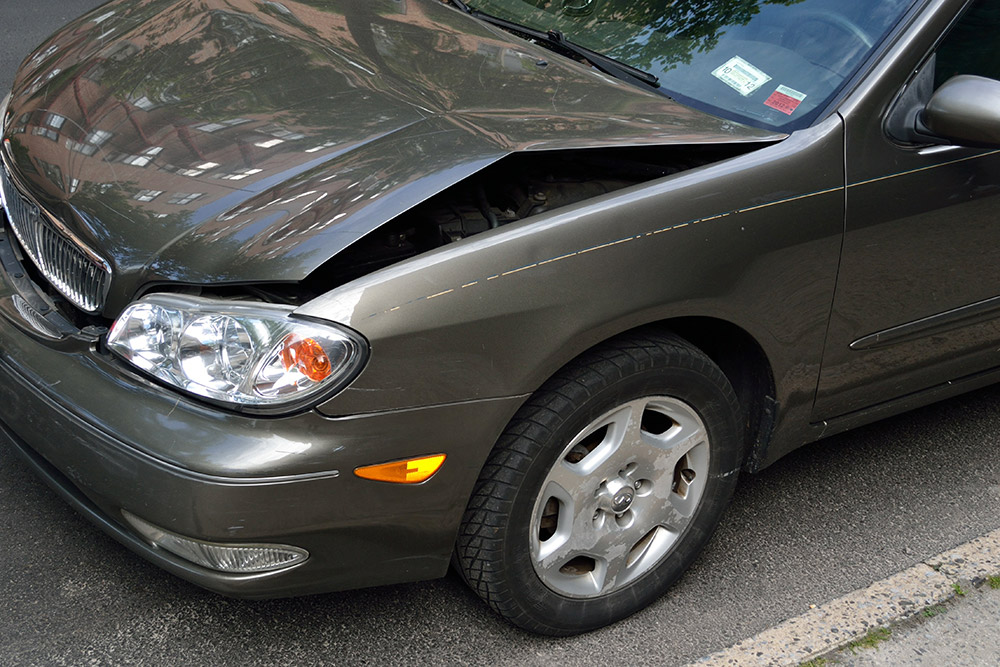
Provided by Artistic Operations/Pixabay
A phone buzzes and a driver reaches to see the text, the car veers out of the lane momentarily, but the driver recovers focus this time. It’s common to see signs of distracted driving like this, where one split second can make the difference between a near miss and an accident.
Impaired driving might result from texting, conversing, eating, having a heavy cognitive load, or acute impairment due to intoxicants or a medical condition. Regardless of the cause of impairment, they all pose a danger, not just to the driver, but also to occupants, surrounding walkers, bicyclists, and vehicles. In fact, an average of 28 percent of traffic fatalities are associated with distracted driving events like these.
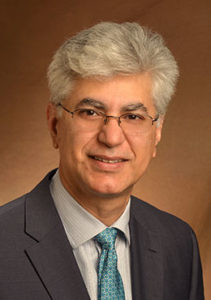
Asad Khattak
A new collaborative research project funded by the Collaborative Sciences Center for Road Safety and led by Beaman Professor Asad Khattak focuses on enhancing driver safety through experiments that identify and detect driver impairment. The project involves students from CEE, the Department of Mechanical, Aerospace, and Biomedical Engineering, and the Data Science and Engineering program at the UT-ORNL Bredesen Center for Interdisciplinary Research and Graduate Education.
“Serving the societal aims of safe mobility, we are exploring the use of big data and artificial intelligence together to find the proverbial ‘silver bullet’ that will better detect driver impairment and enhance safety through a comprehensive analysis of driving volatility,” said Khattak. “Streams of biometric, vehicle, and roadway data will be processed to identify anomalies and actionable strategies in real-time. While this can be a daunting challenge, we hope to overcome it through new surveillance technologies, use of computational resources, and naturalistic data analysis, which will give us leading indicators of crashes. The appropriate use of these data and artificial intelligence can help prevent costly crashes in new ways through digital innovations.”
The research aims to collect multi-dimensional big data to find the correlation of multiple factors with impaired driving and incorporate the related contributing factors to monitor driver behavior and detect impairment. One of the outcomes will be an intelligent Advanced Driver Assist System, which will provide useful and accurate feedback to drivers as well as take evasive action in hazardous conditions, if needed.
To start, the team is conducting experiments in a simulated environment where participants are asked to emulate specific distracted driving behaviors (e.g., texting, reading, looking at scenery, drowsiness, drinking, etc.). They are using a multimodal approach to data collection, monitoring, and analysis to include driver biometric signals, vehicle dynamics and telemetry, and external environmental conditions such as traffic, weather, and day and night conditions.
In addition to data collection, the team is working to develop a model to detect distracted driving by analyzing the streams of data and identifying any abnormality in the vehicle kinematics, driver’s biometrics, and surrounding environment. They are also cleaning data and setting baseline measurements to account for the unique driving styles and biometrics and vehicle characteristics.
This project aims to create a framework and model developed to detect impaired driving and any abnormality in the driver, vehicle, and roadway or environmental system. The resulting AI-based model will be able to be used by the private sector stakeholders in automated and conventional vehicles to reduce the probability of crashes.